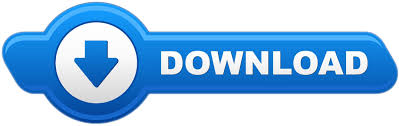

This is fundamental because a marginal effect of a continuous variable is a derivative, whereas a marginal effect of a discrete variable is the change of the Expression evaluated at each value of the discrete covariate relative to the Expression evaluated at the base or reference level. When margins computes an effect, it distinguishes between continuous and discrete variables. This is usually the default prediction (in this case, the conditional probability), but it can be any other prediction available for the estimator or any function of the coefficients, as we will see shortly. Next, we see the concept of an Expression. The first part of the margins output states the statistic it is going to compute, in this case, the average marginal effect. Note: dy/dx for factor levels is the discrete change from the base level. : education percentile 1.divorce 2.divorce 1.pdivorce The average of the change in the probability of being married when the levels of the covariates change is given byĪverage marginal effects Number of obs = 5,000ĭy/dx w.r.t. Probit married c.education#c.percentile c.education#i.divorce /// In other words, an average marginal effect of an interaction between two continuous variables. The average of the change in the probability of being married when the interaction of percentile and education changes.In other words, an average marginal effect of an interaction between two discrete variables. The average of the change in the probability of being married when the interaction of divorce and pdivorce changes.In other words, an average marginal effect of an interaction between a continuous and a discrete variable. The average of the change in the probability of being married when the interaction of divorce and education changes.In other words, the average marginal effect of each covariate. The average of the change in the probability of being married when each covariate changes.I am interested in modeling for individuals the probability of being married ( married) as a function of years of schooling ( education), the percentile of income distribution to which they belong ( percentile), the number of times they have been divorced ( divorce), and whether their parents are divorced ( pdivorce). Using margins to obtain the effects I am interested in This example used probit, but most of Stata’s estimation commands allow the use of factor variables. Factor-variable notation allows Stata to identify interactions and to distinguish between discrete and continuous variables to obtain correct marginal effects. As we will see below, convenience is not the only reason to use factor-variable notation. I did not need to create dummy variables, interaction terms, or polynomials. The result of what I typed would look like this: Stata understands that c.x#c.x is the square of \(x\) and that c.x#i.d corresponds to the variables \(x\) and \(d\) and their interaction. I do not need to create variables for the polynomial or for the interactions between the continuous variable \(x\) and the different levels of \(d\). Where \(y\) is a binary outcome, \(d\) is a discrete variable that takes on four values, \(x\) is a continuous variable, and \(P(y|x,d)\) is the probability of my outcome conditional on covariates. Suppose I want to use probit to estimate the parameters of the relationship The results I am after are not trivial, but obtaining what I want using margins, marginsplot, and factor-variable notation is straightforward.ĭo not create dummy variables, interaction terms, or polynomials I want to estimate, graph, and interpret the effects of nonlinear models with interactions of continuous and discrete variables.
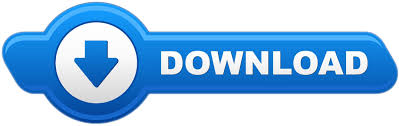